Our first tentative steps towards embracing AI in everyday life (digital assistants, connected devices) are only the beginning. AI in the cloud is like a single intelligence; edge computing – multiple devices all equipped with machine learning capabilities – will be what brings the technology to life in the hands of the average end user. As edge computing becomes more common, and more affordable, the potential use cases are limited only by our imaginations.
Edge vs cloud: the future of AI?
Ironically, the term edge computing was coined and became apparent with the advent of the cloud technology. Long before “the cloud” existed, local and embedded processors helped the industry to evolve into what today we call Industry 3.0. That started in the seventies.
So, it is not that edge processing did not exist at all before the advent of “the cloud” or the IIOT but it was more limited in scope and control capabilities. It was also using traditional Von Neumann type processors (i.e. one instruction at a time), implementing classical, often static, non-adaptive control algorithms.
What we call today “edge processing” is the natural progression of the existing embedded control systems updated with modern embedded processors that have not only more processing power than their 20 century counterparts, but that are also capable of implementing distributed and adaptive processing. That, coupled with the modern communications interfaces (i.e. cellular, LPN, even satellite) and of course, the centralized servers, brought Industry 4.0, starting around 2010, when we talk in terms of “edge” and “the cloud”.
So, what is “the edge”? It is a generic term that describes the extensive number of embedded controllers that are in use today. From inconspicuous embedded controllers existing in any and all industries, to the more glamorous personal communication devices (i.e. mobile phones) to self-driving cars, all are part of the big edge processing family.
...it is not that edge processing did not exist at all before the advent of “the cloud” or the IIOT but it was more limited in scope and control capabilities.
What is edge computing, how does it differ from the cloud, and why does it matter for AI?
Today's edge computing takes advantage of the advancements of low power processors (i.e. i.MX8M Plus) that are not so “low-computational-power” anymore. It can do a lot of number crunching and even perform machine learning functions. A couple of advantages arise: A) Because the processing happens on the edge, the workload of the centralized systems is lowered, and B) Because only the results of that processing gets sent from the edge to the cloud, edge computing also reduces bandwidth requirements. For example, instead of a security camera sending all its images to the cloud for processing, it would send only those images that show proof that an identified event occurred. Although the processing power on the edge today is still much smaller than what is possible on the cloud, edge computing nonetheless has the potential to increase processing power by an exponential amount, by the sheer number of edge computing nodes, if nothing else. As the number of intelligent edge devices increases, our ability to perform edge, real-time AI type processing increases as well.
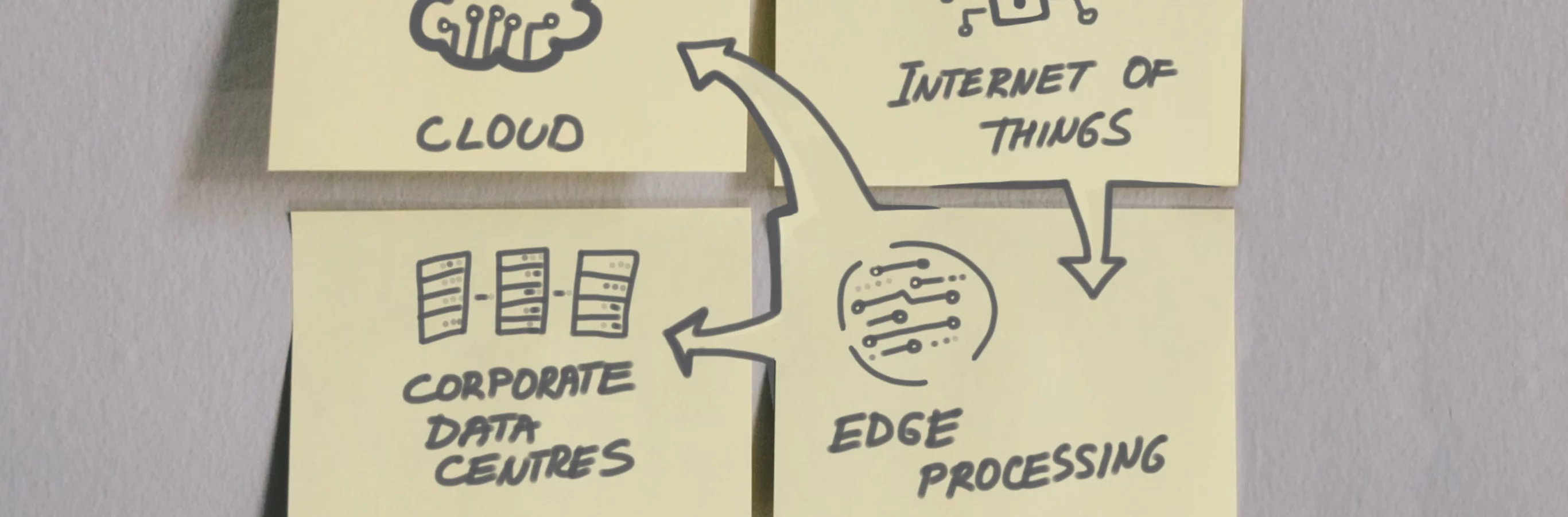
AI isn’t the future – it’s already here
Most of us already use AI daily, even if we aren’t aware of it. Every time we rely on anti-virus software to keep our devices secure, get an Amazon Prime™ or Netflix™ recommendation about what to watch next, or use a grammar checker when we type, we’re using functions that are powered by cloud-based AI. And don’t forget about the “digital assistant” – whether it’s Alexa®, Cortana®, or Siri®, these digital aides help us perform many common tasks, and in doing so, they interface with many other smart devices. So, how does edge computing make this better?
Our mobile phones are an easy example. In many ways, edge computing takes us back to the way things were prior to the Google™ Everything days, but instead of struggling with how your phone’s limited-feature chip misinterprets your voice commands, edge computing now puts the power of AI in your hand. The ability to unlock your phone with a face ID is one common example. Edge computing also has huge applications in photography. Every time your phone automatically adjusts its settings when you’re taking a portrait, or adjusts the light levels when you’re shooting at night, or even fixes a blurry image, that’s because of technology that’s at work in your device, running sophisticated AI-powered algorithms locally.
Edge computing makes AI more practical
As mentioned before, Von Neumann type computer processors work in a linear manner: the first task must be completed, then the next, and so on and so forth. In contrast, artificial intelligence mimics the way our brains function, with separate processing nodes, albeit less powerfully, all working in parallel to deliver a result in short amount of time. Until recently, running AI processes required a lot of processing power, which meant lots of hardware and high energy use. But with today’s edge computing devices, those problems disappear. For example, dedicated edge AI chips (i.e. NDP100) are small, use much less power, and generate little heat. On top of everything because edge computing allows many AI processes to work in parallel, our AI capabilities are enhanced at the macro model as well.
Until recently, running AI processes required a lot of processing power, which meant lots of hardware and high energy use. But with today’s edge computing devices, those problems disappear.
Devices on the edge
We’ve already seen how edge computing allows for real-time processing and analytics of video surveillance, but real-time processing is essential in many industries that depend on nearly-instantaneous analysis of sensor data. When employed in wearable devices, for example, this has transformed the delivery of health care. When used in remote sensors as part of the IIoT, it has the power to minimize equipment downtime and keep workers safe.
Edge AI will also transform the way we use our smartphones. In addition to manipulating and enhancing photos, with edge computing, we’ll talk to our devices rather than tapping a screen. It will enable real-time language translation, and device security will be assured with biometric scans. Augmented and virtual reality will also become commonplace, allowing us to interact with the world around us in amazing ways. Finally, edge AI and real-time processing are the missing pieces to making self-driving cars a reality: this is something that can only happen when vehicles have the near-instantaneous ability to assess sensor data and act upon it.
What about security?
When data is analyzed and processed locally, the implicit risks of sending it back to the cloud are minimized. Short communications are harder to be intercepted as well. Of course, devices must be kept secure and as more companies implement secure communications, the security risks associated with edge computing (already small) will decrease even further. Ironically, if implemented properly, IIoT transactions can be more secure than your day-to-day banking transactions due to the fast pace of technology evolution.